Showing:
— A presently elevated growth rate of CO2 in the atmosphere directly linked to globalization.
— And resulting likely 1.5 degree C warming by 2030, TEN years earlier than the recent IPCC estimate.
— Plus a fascinating story of diagnostic data science discovery.
Yes, it is a somewhat radical approach,
but is fully data driven, meticulous, and at the high side of the IPCC uncertainties, making it plausible. So it should challenge others to try to confirm or dispute the findings.
Losing 10 years in preparing for 1.5 degrees C also makes this finding, if true, extremely urgent to respond to.
(A Major Edit of a 10/8/18 version, republished 4/8/19 – Jessie Henshaw)
The Path of Atmospheric CO2 – To understand climate change it helps to start with the whole picture, the great sweep of increasing concentration of CO2 in the atmosphere shown in Figure 1, as the main cause of the greenhouse effect. Looking at where it began, you can clearly see the fairly abrupt shift in the trends at about 1780, also about the same time as rapid industrial growth was beginning, seeming to mark the abrupt emergence of fossil fuel industry that the rest of the curve clearly represents.
Look closely at the relatively lazy shapes of pre 1780 variation in CO2 back to 1500 (purple) and how that pattern differs from the abrupt start of the growing rates of increase (green line) after 1780 an how closely it follows the mathematical average growth rate curve (dotted). Note how the trendline threads through the fluctuations in the data starting from 1780. The way the data moves back and forth *centered on the constant growth curve* is what implies that the organization of the economy for using fossil fuels had an constant growth rate, of 1.48 %/yr. Hopefully that seems rather remarkable to you, but the data is clear, that the global economy has a single organization for behaving as a whole, as a natural system, with a stable state of self-organization in that period.
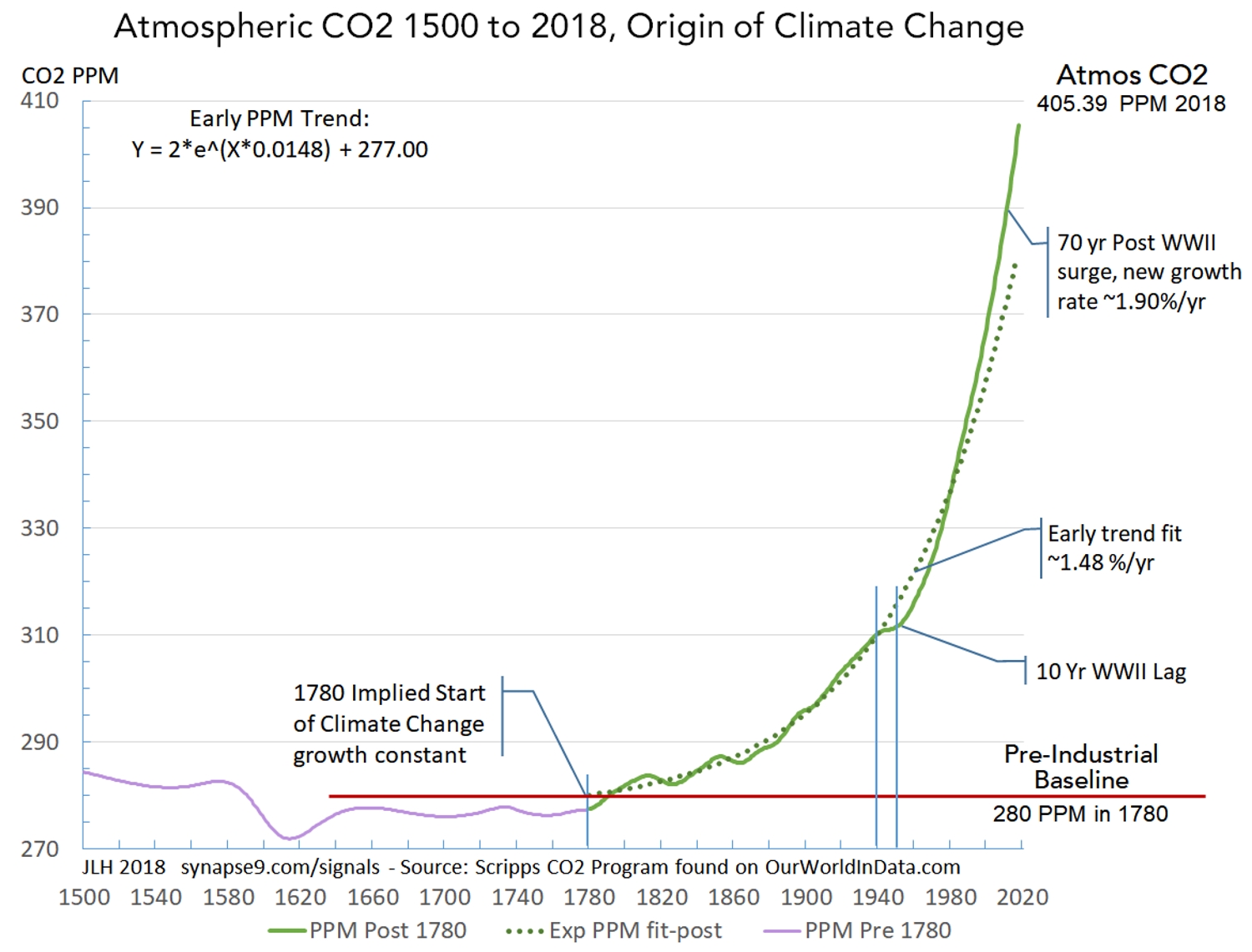
We know from the absorption of heat radiation by CO2, creating the greenhouse effect, that the CO2 greenhouse effect is heating the earth in relation to its concentration in the atmosphere. What implies that relation is close to linear, making the effect directly proportional to the cause, is shown in the Figure 2. The dashed brown line shows the slope of the relation, closely fitting the actual gradual curve, at least between 300 to 400 PPM, the thresholds that were crossed in 1914 and 2016 respectively, a period of 102 years. Atmospheric CO2 is increasing much faster now, though, so the next increase of 100 PPM, to 500 PPM, will be reached much more quickly rising at its current stable rate of 1.9 %/yr rate. If that rate continues 500 PPM will be reached in only 30 more years, by 2046. That large acceleration is the effect of the current higher exponential rate of increase. Of course, considering the rapid compound acceleration of the cause of climate change, and the alarm people are taking now, quite a lot could happen before 2046.
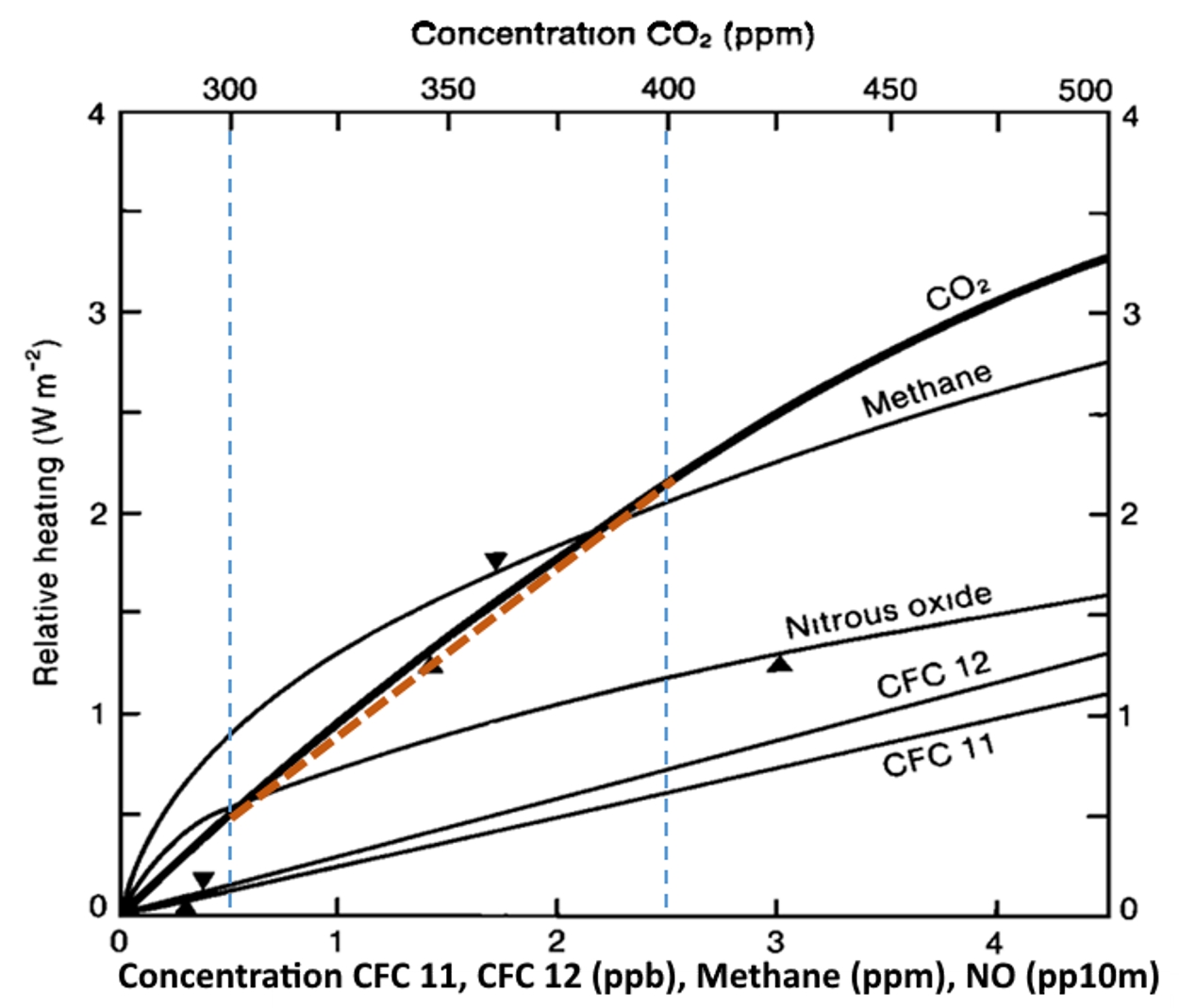
The Annual PPM Growth Rates – Figure 3 shows the growth of Atmospheric CO2 (green) with the details of its fluctuating annual growth rates, to depict both the constants of the growth curve and it’s irregular growth rate interruptions. The individual interruptions raise lots of interesting questions, but perhaps the most important feature is that they are quiet temporary, as evidence of the constant behavior recovering again and again.
The upper curve shows fluctuating annual growth rates (lt. axis, PPM dy/Y) for the curve below, the CO2 PPM concentrations. The peaks and drops of the growth rate align with the small waves in the concentration (rt. axis). Note that the large drops in the growth rate that seem to snap right back to the the horizontal dashed red lines. That seems to show that they mark processes that absorb and then release CO2 again, as they do not seem to affect the average growth rates of PPM concentrations as a whole, around which the annual fluctuations homeostatically fluctuate.
This diagnostic approach is for raising questions like the above, using the annual growth rate to expose the dynamics of the curve for a somewhat anatomical picture. In this case it’s of the homing dynamics of the global growth system as it first hovers around the rate 1.48 %/yr from 1780 up to WWII, and then shifts to hovering around the higher rate of 1.9 %/yr as it stabilizes from 1971 to 2018. You might think of these two long periods of homeostatic growth rates in CO2 concentration as representing periods of regularity in the causal systems, global economic growth and the carbon cycle response, seen through the lens of atmospheric CO2.
You might think the large departures from the regular trends would be great recessions perhaps, that then “make up for lost time” on recovery. I could not find corresponding recessions, though, and for the great recessions I checked there do not seem to be notable dips in CO2 accumulation. To validate this kind of research one has to go through that kind of thought process for every bump on the curve, either a tedious or exciting hunt for plausible causes than then check out with other data.
What seems most unusual about the big dips in the CO2 growth rate (D1, 2, 3, 4, 5) is that 1) they do not occur after WWII and 2) they rise and fall so sharply and have no lasting effect, seemingly temperature sensitive as well as absorbing CO2 later released. I can’t say whether it is feasible or not, but something like vast ocean plankton blooms might have that effect, absorbing and then releasing large amounts of CO2. There’s also a chance the way the raw data was splined and the growth rates smoothed, to turn irregularly spaced measures into smooth curves, might also have unexpected effects. Whatever phenomenon causing the big dips was, it appears to have been interrupted by the rapid acceleration of warming that followed WWII, as evident in the smooth and uninterrupted rise in most recent and best raw data. Those are at least pieces of the puzzle that might help someone else narrow it down.
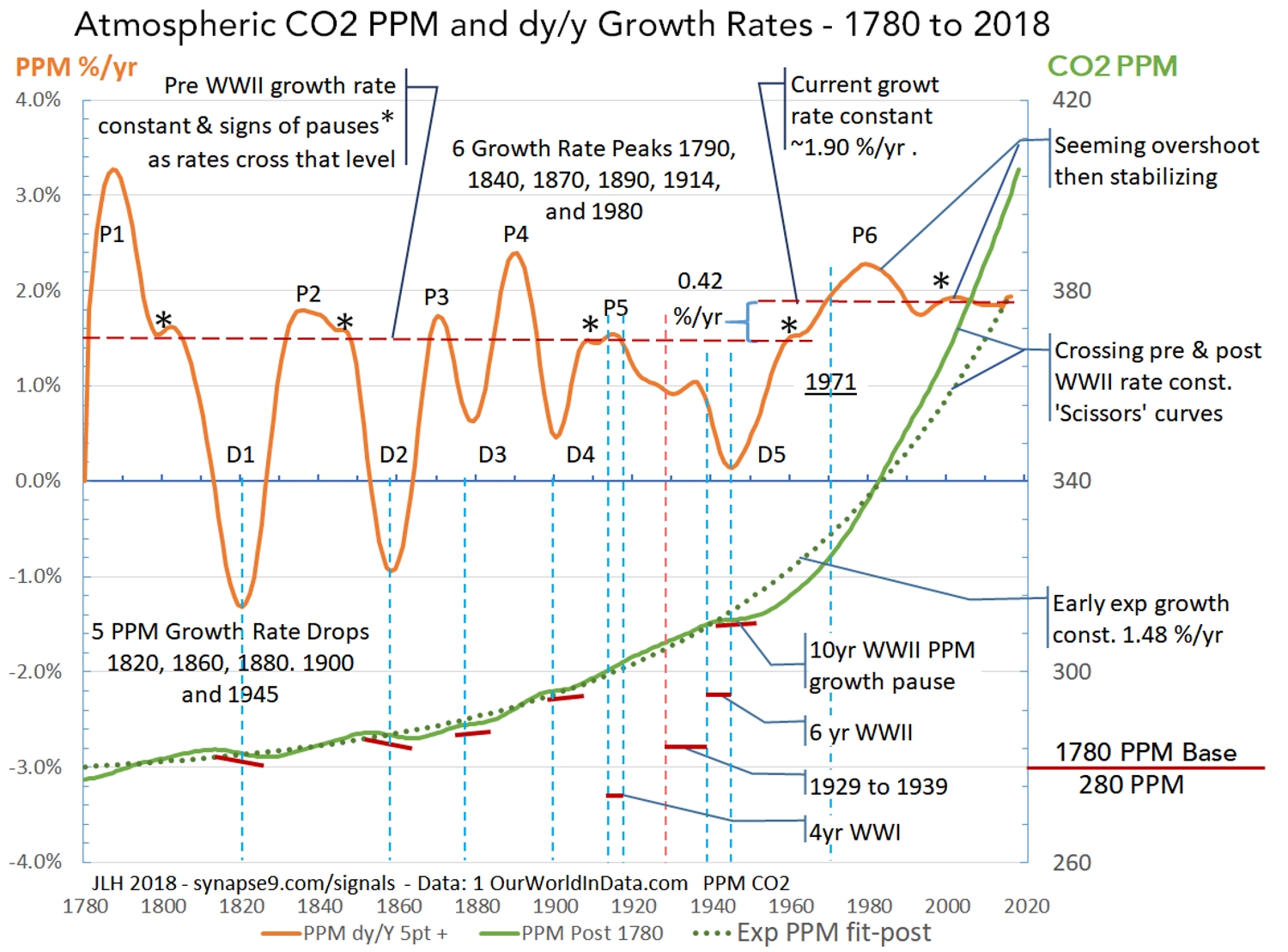
Comparing the CO2 cause and degree C effect – The main purpose of Figure 4 is to compare the history of earth temperatures (blue, ‘C, lt scale) with the curve of atmospheric CO2 (green, PPM, rt scale). The CO2 PPM data is the same Scripps atmospheric CO2 data and scale we’ve seen below. The temperature data is from the HadCRUT4 records used by the IPCC. In this case the original anomaly data relative to the 1850-1900 average have been converted to absolute ‘C values, using a conditional set point of 14.6 ‘C in 2017. In a way it is as arbitrary a coordinating value as the others people use. It’s chosen here first for being a more familiar scale, but also so that 1780 initial values for PPM and ‘C can be determined as initial values for the greenhouse effect. Those baselines are essential for defining the exponential growth rates of the PPM and ‘C curves. The 14.6 ‘C value was based on an expert’s estimate.
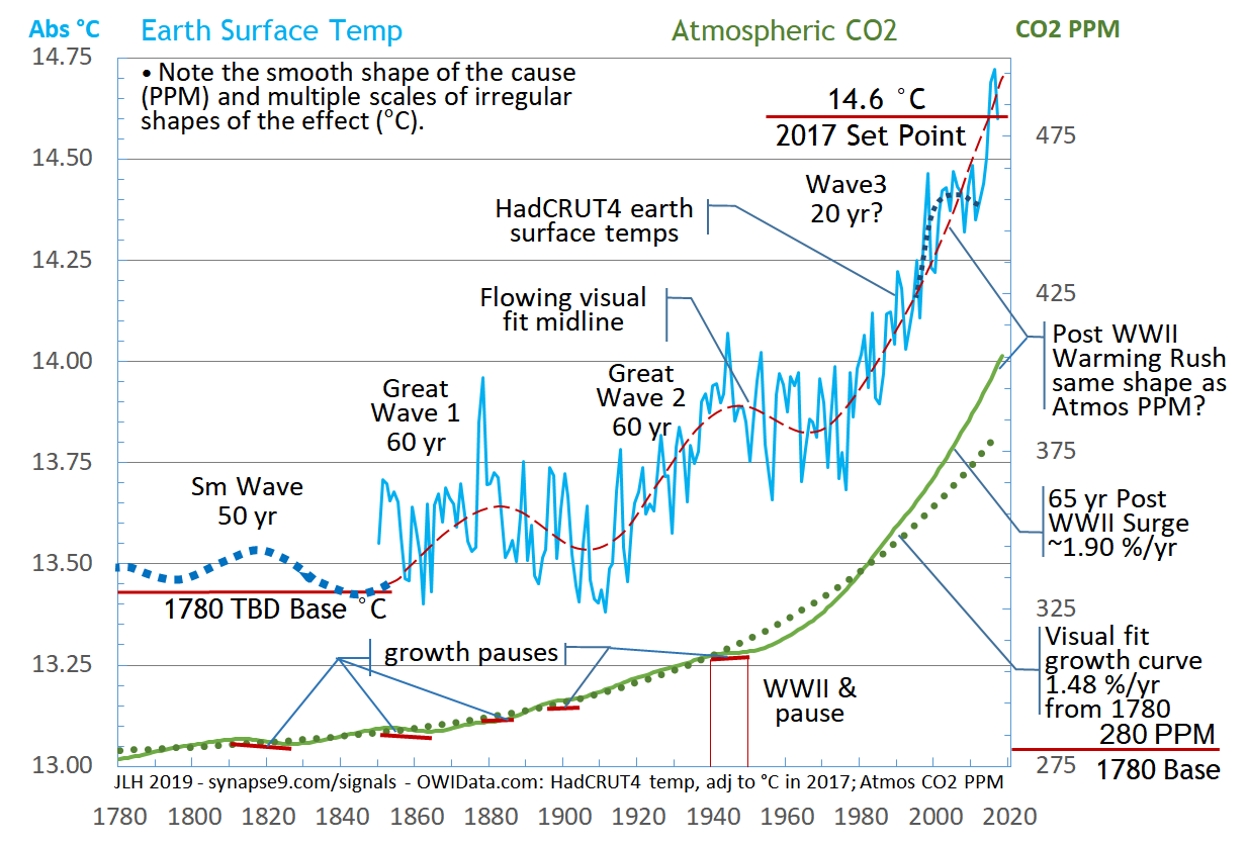
Aligning the curves for Figure 4 lets us look closely to see if any shapes of the cause of the greenhouse effect (PPM) are clearly visible in the shape of the effect, global warming (‘C). Does anything in particular jump out? First might be the differences, one curve quite smooth the other jittery, both having wavy fluctuation patterns too, but of very different scales and periods. The first thing you might ask about is how regularly irregular the ‘C curve is seems to be. That variation is thought to be mostly due to annually shifting ocean currents, along with weather system changes and the difficulty of measuring the temperature of a complex varying world.
The ‘C curve (Figure 4) also shows the two ‘Great waves’ (#1 and #2) in earth temperature that appear to be independent of the greenhouse effect. The dotted red line was visually interpolated as the midline of the irregular but seemingly quite constant fluctuating annual temperatures of the HadCRUT4 data. The blue dotted line was added to suggest earlier large waves in earth temperature copied from the shapes in the ancient temperature reconstructions seen in Figure 5. I physically overlaid those reconstructions of ancient temperatures on Figure 4, drawing a continuation of the Figure 4 midline curve that fit the Figure 5 curves.
One might say the minima of the great waves in the ‘C curve display a trend somewhat like the general trend of the PPM curve, say from 1780 to 1980. The one shape that makes the two curves seem really connected, though, is the way the sharply rising PPM curve (the implied cause) and ‘C curve (the implied response) both start following a “hockey stick shape” in the 1980s. It even seems the shape of the ‘C curve interrupts the great waves as it takes off exponentially, breaking a rhythm that seems to go back many centuries. There is a possibility that the great waves represent upper atmosphere standing convection patterns waxing and waning, something that increasing convection intensity could interrupt. Perhaps that would help others find what the great wave cycle, or not. Since theory suggests the trends of both cause and effect have a linear component Figure 6 shows a linear scaling of the PPM curve to see if it and the ‘C curve can fit.
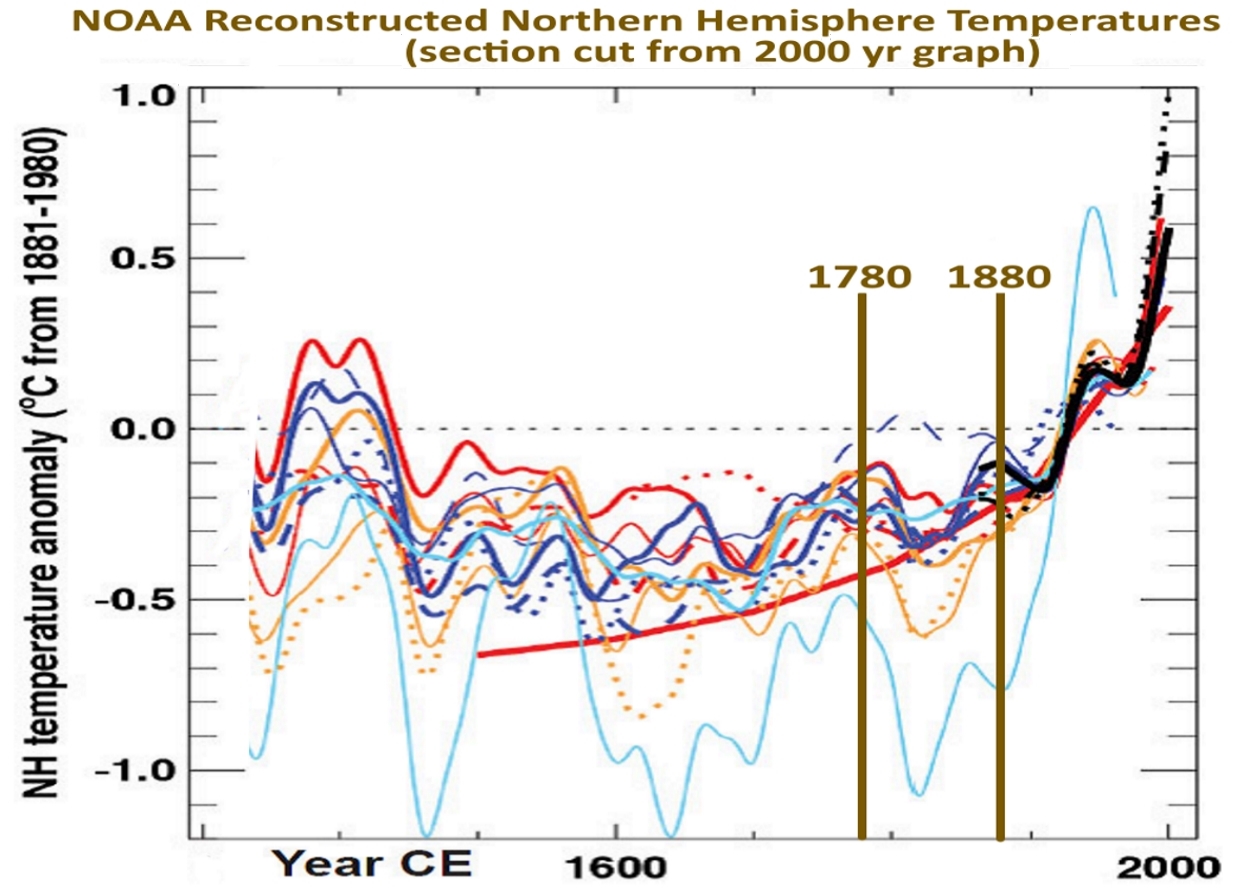
Scaling CO2 PPM to Make a ‘C Proxy – The reason to scale PPM to emulate the dynamics of ‘C curve is simple. The ‘C fluctuation is so erratic the variety of curves to predict its future is rather extreme, so people have been generally using a straight line. An exponential curve is not a straight line, though. So the quite regular shapes of the PPM curve, including its clearly measurable growth constants, 1.48 % before and 1.9% currently, do make it a prime candidate as a useful proxy. Even if the trend has a clear direction now we of course have to allow for increasing uncertainty over time. Adding to that are the plans for dramatically cutting CO2 despite a world economy dramatically increasing its production, a tug of war that could be interrupted by actual war or other economic downturn.
Where the current stable growth rate of climate change seems headed, knowing the PPM curve should be linearly proportional to the greenhouse effect, we experimentally scale CO2 PPM see if it fits the ‘C curve in a logical way (Figure 6).
Scaling the PPM curve to fit the ‘C curve makes a PPM’C proxy curve, hoping to fit the midline of the highly irregular ‘C curve from 1980 to the present. Both the units and the baseline are not determined, though, to produce the proxy curve in PPM’C = A*PPM + B, using a linear scale factor A and a baseline B. A third determinant is then finding a optimal fit between the very different earlier shapes of the curves. So basically I tried lots of things, and found my initial assumptions were mostly wrong. Initially I made the mistake of trying to fit the PPM’C curve to the midline of the earlier ‘Great waves’, and tried several ways until it was clear they were all wrong.
Then I realized those earlier great waves were really not related to the greenhouse effect. So my greenhouse effect projection might better be interpreted as coming up under the earlier systems, like it actually looks. That was purely a graphic device at first. Then when I adjusted the PPM’C curve to pass under the ‘Great Waves’ I set it to go through the miline of local fluctuations instead of the Great Wave departures. Suddenly the fit of rapid growth period became as perfect as I could ask for. I spent some time trying to figure out why, studying all the loose ends, in the end resolving that’s what the data seemed to say. That PPM’C curve then becomes the hypothesized most likely “real” rate of greenhouse effect climate change, and offering a much more narrowly regulated way for projecting its future.
Figure 5 shows both the best fit scaling of the PPM’C proxy curve (dark green dashed line), and its extension to 2050 at its presently stable growth rate of 1.90 %/yr (dashed light green line). Yes there are various uncertainties, but the threat of climate change so far has seemed to be from underestimating, not overestimating, and the findings do appear to be well within the IPCC uncertainties given the difficulty of projecting the temperature data directly.
I think it means that reaching 1.5 ‘C by 2030 is a much more probable estimate of the current trend than reaching 1.5 ‘C by 2040.
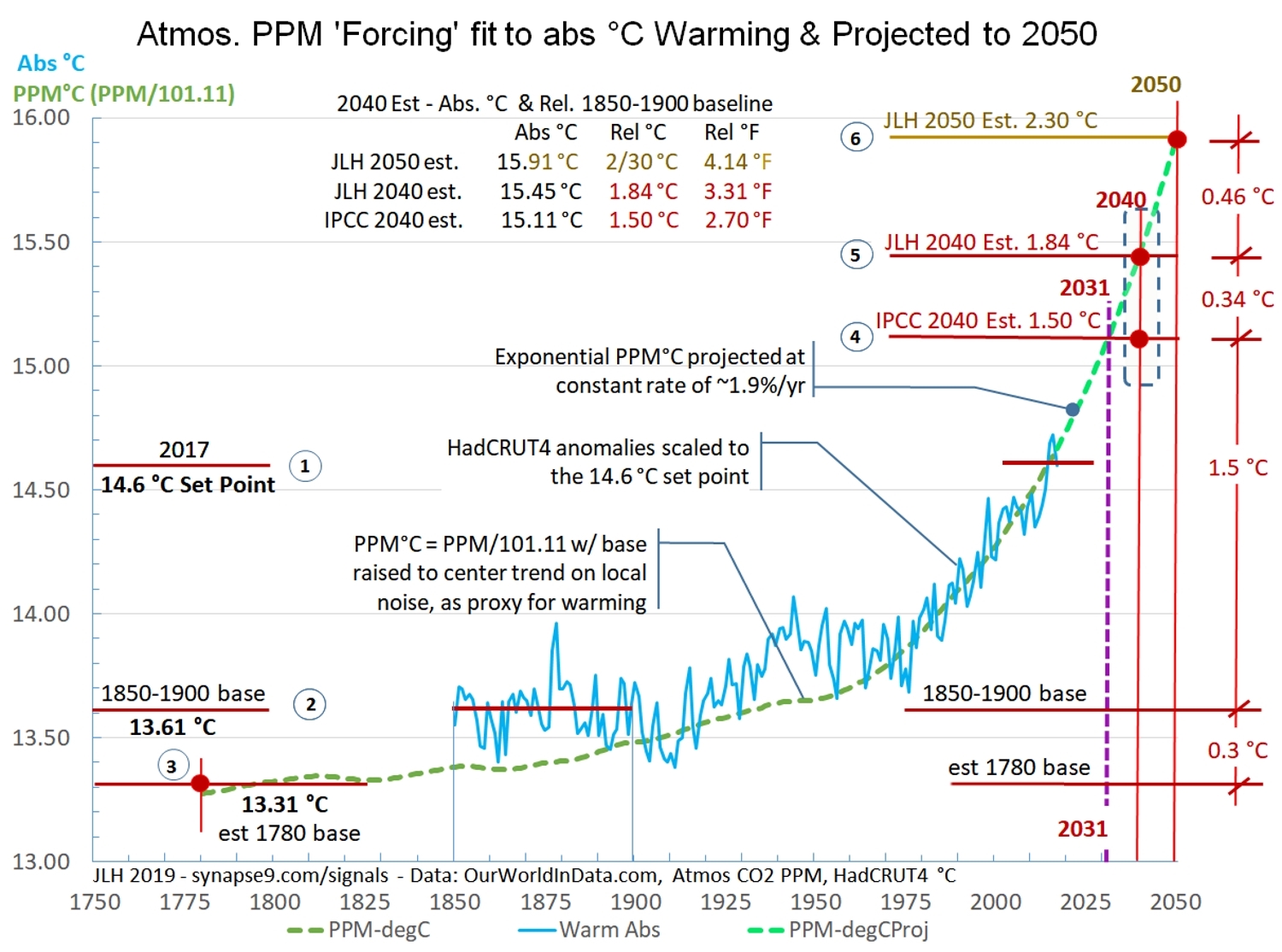
__________________________________________________
The Economy as a Whole – How great a new threat this acceleration in atmospheric CO2 pollution and its greenhouse effect are seems to rest on just how stubborn the global homeostatic regulating systems observed are. That could really change the climate mitigation picture, and help explain why there has been only negative progress in slowing CO2 pollution. So far is seems to have been neglected, with negotiation over mitigating climate change not seeming to take into account the organizational inertia and persistence of the global economic system as a whole.
Figure 7 shows a group of major indicators of the global economy that were selected for having constant growth rates from 1971 (the earliest data for some) to 2016. The GDP PPP curve in trillions of 2016 dollars is growing the fastest, and each of the other curves was indexed to GDP in 1971 in proportion to their relative growth rates. For example, since total economic energy use is growing at about 2/3 the rate of world GDP that variable was scaled to 2/3 of GDP at 1971. This device displays the steady relation between them called “coupling.” That the same proportionality of the growth curves is constant throughout it indicates each of these curves reflects the behavior of the same system. What seems to cement the view that the global economic system appears to be behaving as a whole is the visual evidence that the data of each of these series, like the CO2 PPM data we discussed at length before, seems to fluctuate homeostatically about the growth constant.
What physically coordinates the economy’s coordinated relationships between different sectors displayed here as growth constants seems likely to be cultural constants of each cultural institution, or “silo” of the world economic culture. Every community seems to develop its own expected way for things to work and change and seems to become the way the different sectors end up coordinating their ways of working with each other. That all of this is organized primarily around the use of the exceptionally versatile resource of fossil fuels then indicates that a deeper reorganization of the economy than a swapping of one set of technology for another will be involved. It should suggest to any reader just how very much of the world economy would need to be reorganized, and to be reminded that the last times the world economy was sufficiently disrupted to be reorganized were during WWII an the 1930s.
This topic is also the subject of a longer research paper. Science review drafts are likely to be available later in April 2019.

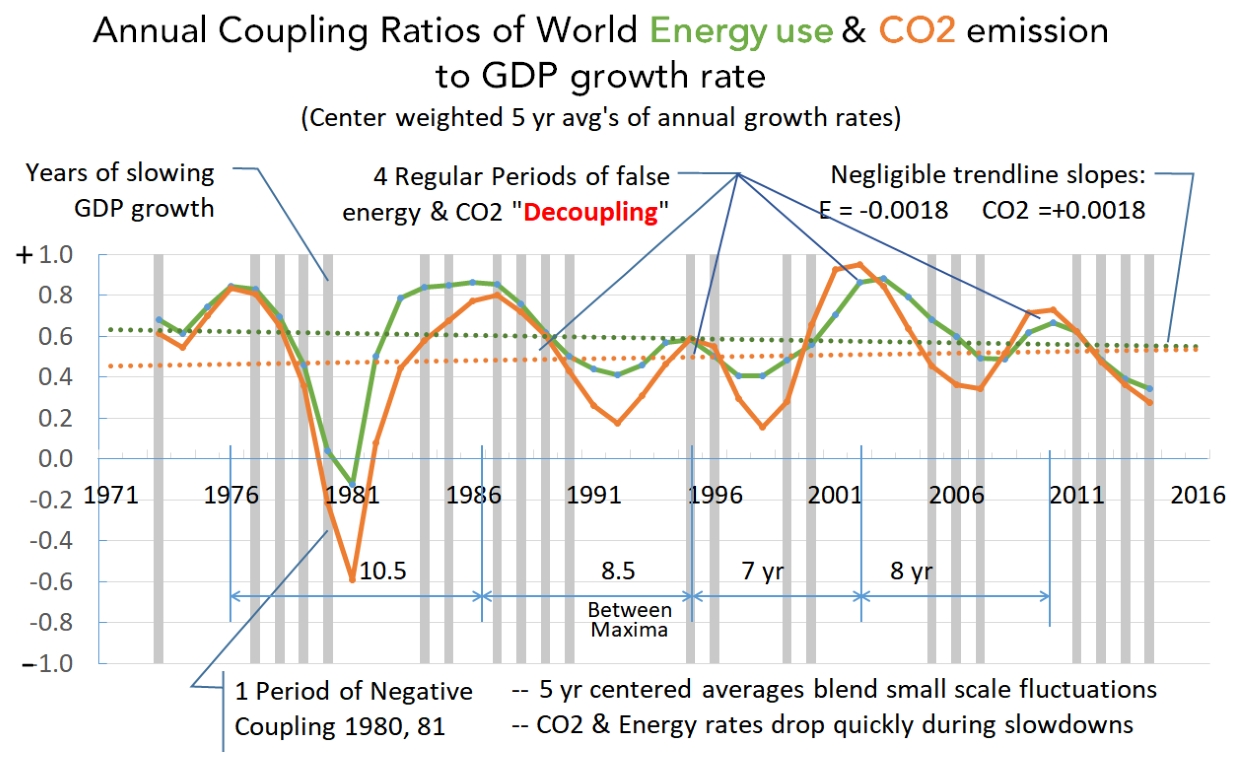
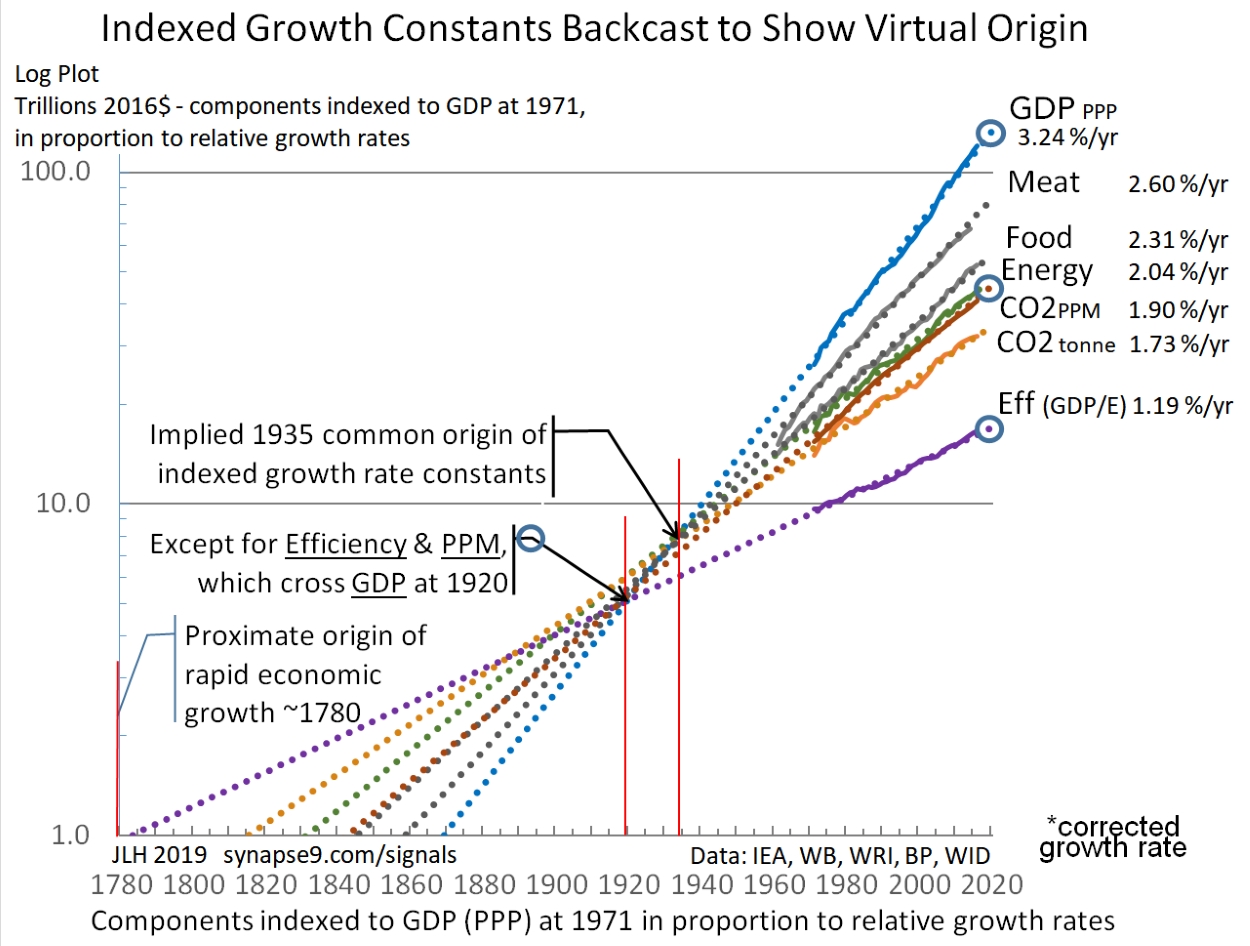
__________________________________
A draft paper Coupling of Growth Constants and Climate Change has full details on the data sources, methods and references:
Data Sources:
- Atmospheric CO2 PPM 1501-2015: OurWorldInData.org
https://ourworldindata.org/co2-and-other-greenhouse-gas-emissions
as well as from the Scripps source directly:
(Scripps, 1958 to present)(Macfarling Meur 2006)
http://scrippsco2.ucsd.edu/data/atmospheric_co2/icecore_merged_products
“Record based on ice core data before 1958, and yearly averages of direct observations from Mauna Loa and the South Pole after and including 1958.” - HadCRUT4 earth temperatures 1850-2017 – Rosner: https://ourworldindata.org/co2-and-other-greenhouse-gas-emissions
- The world bank was my source for GDP PPP and for from 1990 to 2016
– https://data.worldbank.org/indicator/NY.GDP.MKTP.PP.CD?end=2016&start=1990 - World Food Production – 1961-2016 FAO:
http://www.fao.org/faostat/en/#data/QI - World Meat Production – 1961-2016 Rosner – OurWorldInData: https://ourworldindata.org/meat-and-seafood-production-consumption
- Modern CO2 Emissions – 1971-2016, Archived IEA CO2 data extended with WRI CO2 emissions: https://www.wri.org/resources/data-sets/cait-historical-emissions-data-countries-us-states-unfccc
– Because the latest economic CO2 emissions data is 2014 not 2016 as for other data, the trend of atmospheric CO2 was used to project the economic emissions data for the last two missing data points, showing no anomalous direction. https://www.co2.earth/annual-co2 - BP offered energy data in MtOe in its “Statistical Review of World Energy – all data 1965-2017”
– https://www.bp.com/en/global/corporate/energy-economics/statistical-review-of-world-energy/downloads.html - The IEA news item statement that CO2 flattened for 2016 and 2017 was used, just to show how little effect it would have if true
– https://www.iea.org/newsroom/energysnapshots/global-carbon-dioxide-emissions-1980-2016.html
__________________________________________
Work in progress…
Below this line is old text that may be edited in pending updates.
It’s a powerful technique for understanding complex systems, such as the world economy, that behave smoothly as a whole. The most important observation is just that. The system as a whole and these whole system indicators are not separate variables, and the smoothness of the curves shows the system as a whole behaving smoothly as a whole over time.
From our local views of the world that often does not seem to be at issue, though it really is the main force behind all the changes everyone is struggling to adapt to. Individual businesses, cities and countries generally have a quite irregular experience, as their roles in the whole continually change. What the smoothness of the curves and the change in the system as a whole really means is that the world economy is working the just the way it is (financially) supposed to. It is being globally competitive the way money managers manage it, and continually reallocating resources and business to where they will be best utilized, resulting in most every part having somewhat irregular experience to make the whole behave smoothly. The uniformity of these global indicators also says is that their origins all point back to ~1780, when modern economic growth began. We have reasonable measures US economic growth from ~1790, …and so went the world!
Smooth exponential curves and the systems generating them are, of course, among the things of nature with inherent “shelf lives”, relying on systems of developing organization of multiplying scale and complexity, certain to cross thresholds of transformative change. In nature, growth systems generally develop to one of two kinds of transformation, stabilization or destabilization, the crashing of a wave that doesn’t last for example or the thriving business that can last for generations. What characterizes the difference for the emerging systems that last is that, while becoming strong with compound growth (like the systems that don’t last also do), they become responsive and refine their systems to stay strong. In economic terms that’s remaining profit seeking they “internalize their externalities” to mature toward a peak of vitality rather then failure. It’s a choice made in mid-stream.
Understanding what will make that difference in outcome for our global growth system will partly come from people getting a better understanding of how we got here, as shown in the Figures 1, 2, 3 & 4. The growth of technological civilization relies on ambition, creativity and resources, and methods that we could potentially change. How economic growth is largely managed by the application of business profits to multiplying business developments, what makes GDP to grow. If our decisions were to internalize our externalities that is also one of the things that might change, without really changing human ambitions, creativity or resources.
__________________________
JLH
Malcolm,
Thanks very much for picking out some of the tantalizing irregularities in otherwise smooth data. Perhaps you could tell me how the spline used to smooth the ice core Scripps data, up to 1957, could have produced such realistic looking fluctuations from such sparse data points in the early years. I did a study comparing the spline with the raw data, (https://synapse9.com/_pub/warm/Worldc-Long-PPMrateDat-1750.jpg) which shows the differences clearly. Does this kind of spline set limits to higher derivatives as it reconstructs a logical path somewhat near the data points? My systems thinking is up to date, but my math isn’t.
I indeed think the reduction of long wave temperature fluctuations after WWII is due to an amplifying feedback stretching the overall curve, I think maybe caused by the systemization of maximum growth rates for globalization. The acceleration could also reflect carbon sinks being exhausted and new CO2 sources emerging in the globalization process too. The disappearance of the “great waves” is another contributor. My guess on why those largely disappeared after WWII is that the great waves ~60 years apart are due to ebb and flow of upper atmosphere standing convection cells. I have no evidence of that at all, just process of elimination, and seeing the infrared images from space showing lumpy standing convection cells that might possibly have long term ebb and flow dynamics, directly affecting the earth’s temperature. http://synapse9.com/_pub/warm/Earth-Outgoing.jpg I’m using only the HadCRUT4 earth temperature data, so I don’t know if any of the polar differences are reflected, or the ocean conveyor pauses that might be occurring, but after WWII the annual fluctuation pattern seems to be very regular, except for “little wave 3” in the 2000s that sort of coincides with the “great denial” political discussion.
Thanks for the link. Can you suggest how I might get some of my findings into the discussion, or suggest a journal that might publish the associated research paper (a draft is linked at the bottom of this report). I think it would be important to get comment on my way of concluding that the 1.9 %/yr growth rate of atmospheric CO2 after WWII implies a similar current acceleration of climate change, something the IPCC seems not to have noticed the importance of as yet.
Thanks so much for a great response!
Jessie