Appendix to " Models Learning Change" - P.F. Henshaw
Feb 2010
References to Add & Prior
Added Notes Cuts from Models Learning
Change
Appendix I.
(sections removed from Sep 2009
draft to be reincorporated perhaps along with continually accumulating notes...)
A. Distinguishing between information and things
It also takes time to understand how it can be meaningful
to discuss physical systems as existing beyond our information, having their
own organization embodied in their own structures, and independent of the logic
and patterns we see in them. If one attempts to refer to features of
natural systems (local organization accumulating around the movement of energy)
beyond what are visible, the question is if they are not visible how do you
then refer to them at all. What is used to connect these apparently
separated worlds of mental and physical systems, and resolve the subject/object
problem their duality presents, is learning. Learning is a heuristic
mental process that directs attention beyond the knowledge of the learner
toward the experience of things in the physical world from which you
learn.
Others have considered that any “subject” is
necessarily limited to our available information, so any “object” needs to be
reduced to information for it to be referred to and meaningfully exist.
This is one way of stating the Copenhagen interpretation of physics and
Heisenberg’s uncertainty principle, to say “if you don’t know about it you
can’t talk about it”. The approach here is the opposite, to consider the
subject of science as studying things of the physical world beyond our
information, by raising good questions about them. We get our questions
from the information we have, from its incompleteness, and by searching for
paths of discovery. That treats the features of the physical world our
information implies to exist, beyond our knowledge, as the real subject of
science. Rather than excluding the physical world from our concerns,
information is used for referring to it with our questions and following the
paths of inquiry we find productive. That way the ‘real world’ of
scientific information (about a virtual physical reality), and the ‘real world’
of physical phenomena (our virtual information constructs of it describe)
become connected. Both then can be considered ‘real’, alternately looking
with one for the other. Questions may abound, but it seems this dual use
of the words is also much more in keeping with normal usage than either
one. People who consider only their theories or beliefs as “real” treat
the physical world ‘virtual’, while others tend to do the opposite, and most
people seem to go back and forth.
This tension between our incomplete information
and understanding the physical systems we are part of that have organization
well beyond our information is also complicated by the two often being
confused. It’s a common habit to represent physical systems as being the
models and explanations we have deduced from our information about them.
We sometimes have little other way to refer to them. The phenomenon of
light seems to be a well proven reality, but is only known by deduction from
other things, for example. We use our explanation as a “stand-in” for it
in our minds, a way to fill in information about the phenomenon wherever that
missing information is needed. Though the phenomenon of light may not
change, lots of other things we treat the same way do, and that becomes a
problem of functional fixation (blind spot) if our information does not change
with them. Substituting explanations for features of human cultures and
environmental relationships is similarly common and useful, but blocks the
learning process by hiding the open questions that might be important to ask as
environments change. To keep explanations from being mistaken for their
subjects would call for use them to separately refer to the open questions that
remain as well as to what we think we know, as by looking through one at the
other. Otherwise there’s a likelihood of confusing the two, substituting
simple but wrong explanations precisely where physical systems have
their most dense organization and unexpected features. Table 1 lists
major worlds of human information and ideas with their corresponding physical
subject, each representing a learning process about organizational processes,
the understanding and the doing or things.
Explanatory Systems
(information worlds)
|
|
 Physical Systems
(behavioral worlds)
|
|
|
|
Perception, Reasoning & Belief
|
|
·
individual learning and experience
|
Physics
|
|
·
forces, fields, mass, energy, forms of
complexity & change
|
Economics
|
|
·
economies, businesses, human behavior
|
Biology
|
|
·
organisms
|
Ecology & Geology
|
|
·
relations between animate and inanimate
parts of environments
|
Sociology & Anthropology
|
|
·
human relationships and cultures
|
Medicine
|
|
·
practice of health care
|
Engineering, Architecture, Technology
|
|
·
practice of design
|
The Arts
|
|
·
practice of crafts
|
Culture, Business, Government
|
|
·
practice of organization
|
Table
1. Short list of mental disciplines with corresponding
physical subjects
Mistaking mental and physical systems might be
less of a problem if the two kinds of organization, mental and physical, were
similar in kind. Mental models and physical systems are remarkably
different, though, such as one being scale dependent and the other not.
It is also interestingly difficult, for example, to determine if what happens
inside natural systems is deterministic as it sometimes appears, or if parts
act in a way that is truly opportunistic as it also sometimes appears, or a
mixture. The more information we have the more it appears we can
determine, as if projecting a state of having limitless information would mean
being able to determine everything. It is also precisely the
interior designs and behaviors of complex systems that are most opaque to
study, and for which so very little can be determined, that do appear to
represent individuals truly behaving opportunistically. It’s as if the
interiors of the more complex natural systems are distinct holes in an
information world made like Swiss Cheese, where natural system organization is
most densely concentrated in what look like voids. If true, the
substitution of simple deterministic explanations to fill these voids of
understanding, about self-organizing and self-animating things, would result in
a particularly large misrepresentation.
In either case correcting the error of letting
your own information become confused with the subject it is about then allows
some comparison, and making the differences between them useful. Failing
to do so makes explanations self-referencing and become just a collection of
conventions instead of information about any other thing at all. If in
addition systems that were opportunistic are then represented as deterministic
what is lost is even the concept of their having their own original behavior and
reactions, learning and internal world. Keeping them mentally separate,
our dual physical and information worlds, may not be automatic or easy but
considering the information as an overlay, is then just a matter of shifting
attention when needed to the unanswered questions underneath.
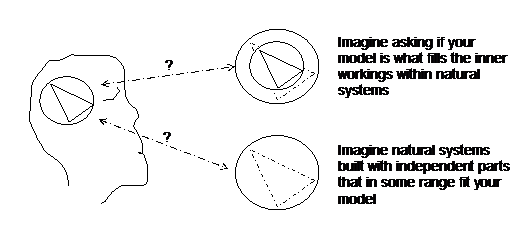
Fig.
1 Are our models what nature uses to operate? If not, does
keeping the two separate help or hinder? Does using models to fill voids
in our understanding of nature hide anything that turning them into questions
would illuminate?
B Why physical things remain undefined
Natural systems, like storms, organisms or
cultures, take care of themselves and work by processes seemingly inside
themselves that remain largely invisible. but One great
difference from our theories is that these and many other kinds of natural
systems appear to be significantly opportunistic in their behavior, as if
probing their environments to seek out new directions to develop. How
that might occur is hidden within their complex and changing forms, making any
line between opportunistic and deterministic behavior unclear.
Having it hard to see how they work does not keep one from watching for warning
signs for when they will change direction though, or find a few reliable
regularities. Watching them change direction helps locate where they are
too, and then some of our considerable native understanding of them that is
part of natural language can be used. The subject of natural systems actually
seems to be the main subject of natural language, one or another way.
There’s hardly any topic of conversation that doesn’t concern one or another
kind of leaderless network of relationships, with real properties and
consequences for us personally. Many common words refer to them and their
general properties. Natural systems are the subject of anything one might
refer to as “established” or “on track” or “off track” or “coming to a
head”. It also refers to natural systems to discuss most anything as
having “relationships” or as “growing” or “developing”.
Natural systems tend to be cohesive, but among
the many reasons it is quite hard to follow how they work is that, like in your
body, the activity and parts of such networks of connection are changing
everywhere all the time. That means that wherever you look nearly all the
real action is located somewhere you’re not looking. Still, with many
kinds of complex natural systems the closer you look you don’t find
chaos. You more often find more scales of exquisitely organized processes
arranged in leaderless networks, with similarly distributed changing
parts. The individuality of natural systems is another aspect of them
that is stubbornly resistant to explanation, the appearance that no two physical
things are ever made alike. That what theoretical constructs are based on
is exact and completely unvarying replication, rigid rules of logic, points to
another basic difference between them.
A number of other scientists studying the
relation between systems of equations and physical systems have also reached
the conclusion that natural systems actually can not be validly modeled, or if
they were their models would be ‘incomputable’. Walter Elsasser (1987)
found that the persistence of diversity in natural form (heterogeneity) would
conflict with the assumption of statistical causation that underlies
mathematical science. Robert Rosen (1991) showed that modeling systems
would at minimum require infinite regressions that no machine could perform and
so nature could not be a machine or modeled by one. Rosen (1996) also
observed that living systems seem to incorporate divergent series of changes
and scientific models can only be usefully defined using convergent
mathematical sequences. In addition various systems scientists such as
John Sterman (2002) have observed that “All models are wrong” for a combination
of causes ranging from natural human failings, to the deep complexity and
profound lack of information about most kinds of complex systems of interest.
These all point to modeling failures as being largely for natural causes, a
difference between the subject and our information.
These observations on the fundamental problems
of why models fail were essentially cautionary, though, rather than providing
improvements on any method for modeling complex systems. Not to diminish
the value of any of the extensive efforts of others in finding regularities
that a mathematical model could be reliably be built around, the approach here
is to identify predictable irregularities, identifying where models are about
to need to change. It could be seen as an application of Rosen’s later
observation, that nature is full of what appear to be divergent sequences of
development, for which useful equations can’t be defined. Looking at what
nature can do that math cannot do, and for what math can do that nature cannot,
provides a way to connect them through the questions about how they differ, and
use each to learn about the other. The method introduced in section
3. uses a family of implied violations of the conservation laws that
physical systems are subject to but mathematical systems are not. They
generate very useful questions about when modeling will need to go beyond the
available information to discover what new assumptions will be needed.
C Connections between natural systems and formal systems.
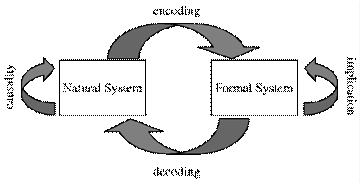
Presented
world
|
Learning
to coordinate systems different in kind
|
Re-presented
world
|
Fig. 2 – Natural &
Formal Systems - Robert Rosen 1991 with added notes
Fig. 2 is Robert Rosen’s diagram of the relation
between natural and formal systems, how the theoretical world is assembled
(encoding) and used to guide us (decoding) in navigating the physical world
. In addition to the difficulties of making good models that Sterman and
others identify, there are some very odd features of physical causality that
models could never be made to emulate. Natural systems are all
individually different and continually changing everywhere at once, with each
part changing differently. Any model that did that would not
compute. Our ability to describe physical things requires using general
categories and statistical uncertainty, but physical subjects are not composed
of categories, and also appear to be completely particular in every detail,
apparently having no ambiguous feature of any kind except for the ambiguity
faced by an observer in how to describe or explain them. Natural systems
also have multiple scales of differently organized parts. Their
multi-scale networks of separately changing parts are held together by
interacting through and with their environments, and so are not at all
self-contained or self-consistent in design. Information about any part
will very predictably omit relevant information about the other scales of
organization around and within it.
Mathematical models rely on fixed definitions
and complete information, though, having none of those odd features of
nature. Mathematical models need to be self-contained and self-consistent
because they have nothing to hold them together but logic and the abstract
definitions the logic connects. Models rely on natural regularities of
unknown origin, but there’s a flaw in relying on them if we don’t understand
something about where and when they might change.
In the process of observing and formalizing
natural systems people reduce nature to information. We record
information by counting individual physical events and the results of periodic
measures grouped in defined categories, using a mix of fairly narrow as well as
broad and loose definitions. Sometimes we keep track of whether the
measures we use are of conserved change in accumulative processes or
non-accumulating indicators, and sometimes not, and represent changes in one as
factors for the other. Our information categories are not naturally
connected, in general, other than how we use them.. Consequently it can
be quite unclear what we are measuring and what it is information about except
the conventions of normal practice. We also may not think that our
categories change, for example, but nature may fill them with all kinds of
changing things, even if we use the exact same procedure for collecting data
for them over and over. For a simple hypothetical example, you may ask a
standard interview question one year, and find that the words have changed
meaning the next, or try to track the price of a standard “market basket of
goods” to find later that no one buys those things anymore. That nature
is not as confused as the arrangement of our information is sometimes only
evident in what Elsasser described as “persistent heterogeneity” or Rosen as
“life”, the evident continuities displayed in our separate categories of
information that seem associated with the continuity of entire systems
beyond our description. A great many of the natural systems displaying
real continuity that concern people are full of independently learning and
responding parts, like economies and ecologies, that are both highly complex
and evidently take care of themselves. Reading those continuities, as
evidence of implied natural systems, and their systematically diverging
relationships as evidence of approaching change helps point to where and how it
will occur, and the need for connected formal explanations, whatever their
arrangement is, to adapt.
Having signs of when they will change would be a
way to study how natural systems are so very effective in taking of themselves,
without controls, clearly displaying methods of very efficient cooperation in
many ways. There is a strong similarity between how neatly the parts of
many kinds of natural systems fit together and provide complementary services
to each other. The wastes and inadequacies of one part often serve as
resources and opportunities to develop for others. If the parts of
natural systems were actually opportunistic, that could well explain that odd
feature. Opportunistic systems would use what they find free to take,
fill in where there are gaps, avoid conflict as a waste of energy. It
would also explain the coincidental similarity between how economic markets
link complementary talents and businesses and the way natural environments are
similarly assembled from complementary parts and functions, if nature’s main
method of creating systems were similar to the way people make their
livings. If so it would provide a new diverse group of physical models of
more successful kinds of economies that don’t build to collapse, for studying
how .
D Reading beyond one’s information
That learning pushes you find ways to read
beyond one’s information, and relate to physical things and relationships that
may remain partly out of view, takes also being cautious to not jump to
conclusions. Hypotheses need some testable foundation. A
“hypothesis” is itself a cautious reading beyond one’s data in search of evidence.
Even representing nature with equations, for example, is by itself a leap of
faith in representing complex physical things with a symbolic construct and
implies a “reading beyond the available data” that we choose to rely on.
Identifying progressions that will be certain to upset the system of relations
they are part of is then just another kind of valuable information about things
presently hidden, an open question with a solid foundation. Discovering
evident gaps in explanations, such as energy densities implied to approach
infinity or zero has led to numerous discoveries of physics. The
discovery of atoms and atomic particles partly resulted from trying to explain
how to avoid unlimited electric field densities.
Still, referring to things that have yet to be
discovered, and are known mainly by something clearly being missing from view,
can easily be confusing. Common language has a way to do it, by just
pointing to undefined physical things with names, For many of the unusual
forms of natural systems and their organization there are as yet no names, nor
a good way to point. What seems clear is that our own very limited
logical constructs for them are clearly organized in a rather different
way. I will propose some terms and uses, but those who prefer to be
cautious might continue to begin from the presumption that individual physical
systems are always undefined and actually contain nothing to directly inform us
themselves. Some others, perhaps the “agnostics” may then wish to follow
that by a ? mark, asking that statement as a question. At the very least
I think it’s it appropriate to always continue to refer to the physical
organization of natural systems hypothetically. What people find inside
the workings of natural systems, say looking inside a human body or trying to
understand what water is, continues to fill books of facts and remain
inexplicable. If what we see from the outside of any locus of
organization has some predictable regularity, we still generally don’t know
quite where it comes from and so may never quite know when it will change.
That we have a natural way of pointing to
natural systems without specifying exactly what is being pointed to at is a
start can be improved on. The existence of their interior designs of many
kinds of natural systems is evident from how they change everywhere at once,
using multiple nested scales of organization, changing scale and form at the
same time with no apparent communication from elsewhere. Something holds
together their great networks of separately developing and adapting parts,
interacting with others through environments with undefined boundaries.
Equations of controlled variables can’t do that and asking why real and formal
systems differ is a way asking productive questions. If one of the main
features of natural systems is their inexplicably complex and smooth operating
interior designs, one place to start is observing that to us they seem
organized around a “wilderness of unknowns”, definite realities that
remain “undefined and unexplored”. Thinking of the “envelope” of all that
is possible to explain, individual natural systems each represent significant
holes..
Nature is an exemplary organizer and builder of
vaguely machine-like systems. The numerous systematic growth processes
that smoothly develop exceptionally complex things with only the process and an
environment of loose parts to account for it is a primary example. That
is so very different from how logically defined and controlled systems operate
that the problem may not be that we have not yet found how natural systems are
organized, but that they may not actually have any of our kind of definable
organization at all. It may not then “explain” them, but just be very
helpful for making models we can trust to be able to tell when natural systems
are changing form and new assumptions for our models will be needed. The
object here is then to define some limited ways to predict when and how our
models will become undefined, and point to learning paths for finding new assumptions
to make and accommodate the natural changes taking place.
E Raising questions of change
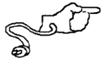
animated glove?
|
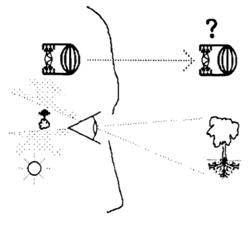
animated world?
|
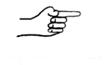
animated hand?
|
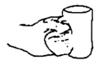
animated cup?
|
Fig
3
Which wholes does one choose to model?
The “null hypothesis” here is that trustworthy theories
reflect some regularity of the complex physical world beyond their information
and so do not contain information about the range of their own validity.
For questions about the limits of their validity someone would need to look
into the gaps in their information. So in order for models to imitate
natural systems and adapt to their environments as natural systems do, models
would need their makers and users to do the learning needed and change
them. What requires the learning is not that modelers are not
proficient in emulating any form of behavior that can be specified. It’s
that we cannot specify behavior beyond our information, particularly if it
concerns natural systems which themselves may be learning processes or
self-animating. If you consider a model (fig. 3) as an animated
glove, designed to emulate the animated hand of nature, it may not contain
information about whether or not the fingers in the glove or the cup held by
the hand are connected.
If the regularities of the physical system taken
to be constant were to change, or “come alive” as it were, then the
assumptions of the model would become invalid leaving the model and perhaps the
purpose it was designed for undefined. Technology change frequently
presents this kind of problem of basic changes in relationships that were once
assumed constant and unexpectedly redefine the meaning of the questions people
have. The changing scale of our economic footprint on the earth does as
well, forcing a general rethinking of the purpose of development and our
relationship with the earth. If you know that’s coming it has the effect
of turning the “=” signs into “?” marks, in time to learn how to change the
model before it fails..
For example, the usual way models of growth and
climax are defined, say in maximizing the use of a limited resource, is with a
logistic curve. That approximates the transition from positive feedback
in seed growth followed by negative feedback in responding to limits with one
continuous equation. Clearly in nature those are often two separate and
distinct processes operating by two very different kinds of systems of
response. In particular, the constraints of natural limits do not begin
with the first use of a seed resource. As a seed resource is uses systems
are self-limited as they develop their ability to use what is plentiful , but
as the demands of the growing system exceed it’s available resources and force
it change what it is responding to. That means that in nature there is a
break in the middle of the sequence where the responses of the physical system
to one thing end and to another thing begin, a discontinuity. That means
it’s possible that any logistic curve mistakenly represents a succession of
different systems as a single system, and skips over the organizational
discontinuity between them. In that case the inflection point in the
response curve from growth to climax possibly needs to be read as a
question mark (
= ?) about what physical
system change the missing discontinuity in the equation represents. The
system starts with quite different boundary conditions than it ends with.
Logically, of course, a system can’t have
information about its end before its beginning, any more than a car fender
would be starting to bend for the tree it will hit as soon as the car heads
toward it. So there must be at least two periods of regularities with
different equations, not one, with new information needed about what will
change to define the transition. What is then clearly missing from
logistic curves is the point or period of time in which changes to the assumed
regularities of the system beyond the information of the model occur and begin
following new boundary conditions.. Therefore some system changing event
occurs, due to change in features of the physical system beyond the information
of the model. They might be either within the system or its parts or in
the environment in which it operates, or both.
Examples abound of natural systems that begin
with unconstrained growth brought to an end by some internal change rather than
some external bound. Organisms generally mature to some optimal size for
their life ahead rather than being limited by exhausting their
environments. Air currents and drips of water are also self-limiting in
how they develop, coming to points of development where they separate from
their sources rather than continuing to develop to the exhaustion of their
sources. Externally limited processes are common as well too, as well as
systems that exhibit a combination of internal responses to external limits,
such as animals foraging and searching for food as they also seek to avoid
danger, or crystal formation or bee hive designs based on modular elements that
are replicated to consume other natural limits.
Often times one can tell from a model that the
development of a system will be limited, though the model won’t say how
exactly. If the change in boundary conditions and behavior in switching
from growth to climax in natural systems takes place due to a combination of
internal and external forces, many of which could involve complex behavioral
systems on other scales, what is happening is more complicated than an
equation. You then have one complex process coming to an end and setting
the stage for the next. What determines how the change will occur is made
by interactions between elements within and around the originating system that
are unprecedented. Studying a model for where these are implied to take
place as if inserting question marks into the models for “what’s happening here
?” then helps locate and discover them. It's "raw science" not
"finished science", and intended as a way to extend either beginning
or advanced research into any field by raising new kinds of focused questions
about changing environments. It's also a way to open our minds and learn
how to follow nature's actual processes.
At first the ability to follow successions for
system states takes time to develop, but we also all have somewhat highly developed skills for it in areas of personal
experience. Many of the same kinds of questions about when
developmental stages will be changing apply very broadly.
Raising children, for example, exposes you to a succession of natural stages of
development that are temporarily stable. As change from one to the next
approaches it exposes threads of new questions and issues that both
parent and child need to follow to be able to make the successions from one
world of relationships to the next. Sometimes such changes catch either
parent or child off guard as circumstances race ahead of preparation for
them. Sometimes they’re prepared for long ahead. The same would apply
in not an entirely dissimilar way to the issues surrounding the maturation of
lots of other kinds of growth systems, a business, a whole civilization, or
single projects that start from scratch like a group of kids learning a school
play. Each of these are of different kinds and on different scales but of
the sequence of developments has many things in common.
|
|
 |
Appendix II. References
A. References to add - 2/4/10
Peter
M. Allen 2010 Editorial, Emergence Complexity & Organization, 11-4 2009
- "In an evolutionary universe, we are destined to continue a perpetual
learning process without end [as does our environment] as we develop, correct
and adapt our interpretive frameworks to gradually fossilization which, fortunately,
we then fail to pass on to the next generation."
TFH
Allen, JA Tainter, J Chris Pres, TW Hoekstra 2001 Dragnet Ecology - "Just
the Facts, Ma'am": The Privilege of Science in a Postmodern World
BioScience, v 61-6
- narrative as the art of science
Brian
Goodman 1994 How the Leopard Changed its Spots. Princeton Univ. Press
W.
Richard Scott 3003 Organizations, rational, natural and open systems, 5th Ed.,
Prentice Hall
B. Prior References
Elsasser,
Walter, 1987. Reflections on a Theory of Organisms - Holism in Biology Johns
Hopkins
- found that the persistence of diversity in natural form (heterogeneity) would
conflict with the assumption of statistical causation that underlies
mathematical science.
Elsasser,
Walter, 1966 Atom and Organism - A new approach to Theoretical Biology,
Princeton Univ. Press.
Henshaw,
P. F., 2010. Complex Systems. Encyclopedia of the Earth
http://www.eoearth.org/article/Complex_systems
Henshaw,
P. F., 2007. Notes - Mathematical tests for punctuated equilibrium in
draft plankton evolution paper “ Flowing processes in a punctuated
species change” http://www.synapse9.com/GTRevis-2007.pdf
Henshaw,
P. F., 1999. Features of derivative
continuity in shape. Journal of Pattern Recognition and Artificial
Intelligence (IJPRAI), Special issue
on Invariants in Pattern Recognition, V13 No 8 1999 1181-1199 http://www.synapse9.com/fdcs-ph99-1.pdf
Henshaw,
P. F., 1985. Directed Opportunity, Directed
Impetus: New tools for investigating autonomous causation. Proceedings;
Society for General Systems Research 1985, Louisville KY http://www.synapse9.com/DirOpp.pdf
Rosen,
R.,1991. Life Itself. Columbia Univ. Press
- showed that modeling systems would at minimum require infinite regressions
that no machine could perform and so nature could not be a machine or modeled
by one
Rosen,
R., 1993 On The Limitations Of Scientific Knowledge, in: Casli, J. L. (Ed.), On
The Limits To Scientific Knowledge. Perseus Books
- also observed that living systems seem to incorporate divergent series of
changes and scientific models can only be usefully defined using convergent
mathematical sequences.
Salthe,
S., 1993. Development and Evolution: Complexity
and Change in Biology, MIT Press.
Sterman,
J.D., 2002. All models are wrong: reflections on becoming a systems scientist.
Jay Wright Forrester Prize Lecture. Syst. Dyn. Rev., 18, 501–531 http://web.mit.edu/jsterman/www/All_Models_Are_Wrong_(SDR).pdf
Appendix III. Added Notes
2/4/10 - Once sorted a bit, none yet...